This section overviews the research fields of the MIC Lab in an illustrative manner. Please refer to the research fields tab for more details.
Consider a ground vehicle equipped with electric drive, automation, and vehicle connectivity, commonly referred to as a Connected, Automated, and Electrified Vehicle (CAEV). The CAEV has the ability autonomously determine its own behavior by utilizing valuable information obtained from vehicle connectivity, including neighboring vehicles' states and real-time traffic conditions. The electric drive system enables the vehicle to move with agility and energy efficiency, making it an ideal solution for future mobility.
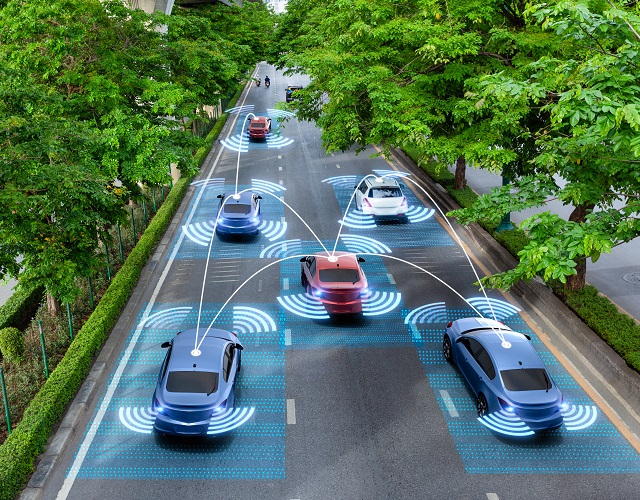
Figure 1. CAEVs
Now, let's imagine you as the user of the CAEV. Your role is simply to provide the destination for your trip. From there, the CAEV must determine the control actions to reach the destination with the best efficiency in terms of energy consumption, travel time, or traffic conditions. This represents the main problem we aim to solve.

Figure 2. Main problem
However, addressing this problem as a whole is incredibly challenging due to its large dimensionality, covering the entire operating time, and the uncertainties associated with future operating conditions.
Therefore, we have broken it down into four distinct subproblems.
The first subproblem involves determining the global behavior of the CAEV. This includes deriving optimal routes and velocity profiles through the application of
"optimal eco-driving control" techniques. By optimizing the driving behavior of the CAEV, we aim to maximize energy efficiency while ensuring smooth and comfortable travel.
In cases where the CAEV incorporates multiple power sources, such as a hybrid electric vehicle (HEV), or multiple energy sources, such as a fuel cell electric vehicle (FCEV), we tackle the trajectory of the power split ratio between these sources through
"optimal energy management" strategies. This subproblem focuses on finding the most efficient utilization of the available power sources to achieve optimal performance and reduce energy consumption.
Figure 3. Optimal eco-driving control & Optimal energy management of a CAEV
Now, let’s consider a future traffic network where the target CAEV operates alongside other CAEVs. Each vehicle has its own planned global behavior to reach its destination. However, conflicts can arise when their planned behaviors intersect in their vicinity. Resolving these conflicts represents the second subproblem. Through the application of
"optimal coordination control" strategies, the CAEVs locally adjust their planned behaviors to facilitate smooth and safe interactions, ensuring harmonious coexistence in the traffic network.

Figure 4. When the optimal coordination of CAEVs is required
The next step is to tackle the third subproblem: controlling the target CAEV to accurately follow the planned local behavior. While this control is generally achievable in normal operating conditions, dynamic operations or unfavorable road conditions can pose challenges. Under such circumstances, the CAEV may struggle to maintain stability and deviate from the planned trajectory. To overcome this, we employ advanced
"electronic stability control" techniques that actively adjust wheel torques to ensure stability and trajectory following even in adverse conditions.

Figure 5. When the electronic stability control of a CAEV is required
CAEVs are propelled by one or more electric machines, making the electronic stability control primarily focused on determining torque commands for these machines. To ensure the effective implementation of electronic stability control, it is crucial to control the electric machines accurately and energy-efficiently, following the desired torque commands. This represents the final subproblem. To address this subproblem, we apply advanced
"optimal torque control" techniques specifically designed for electric machines.
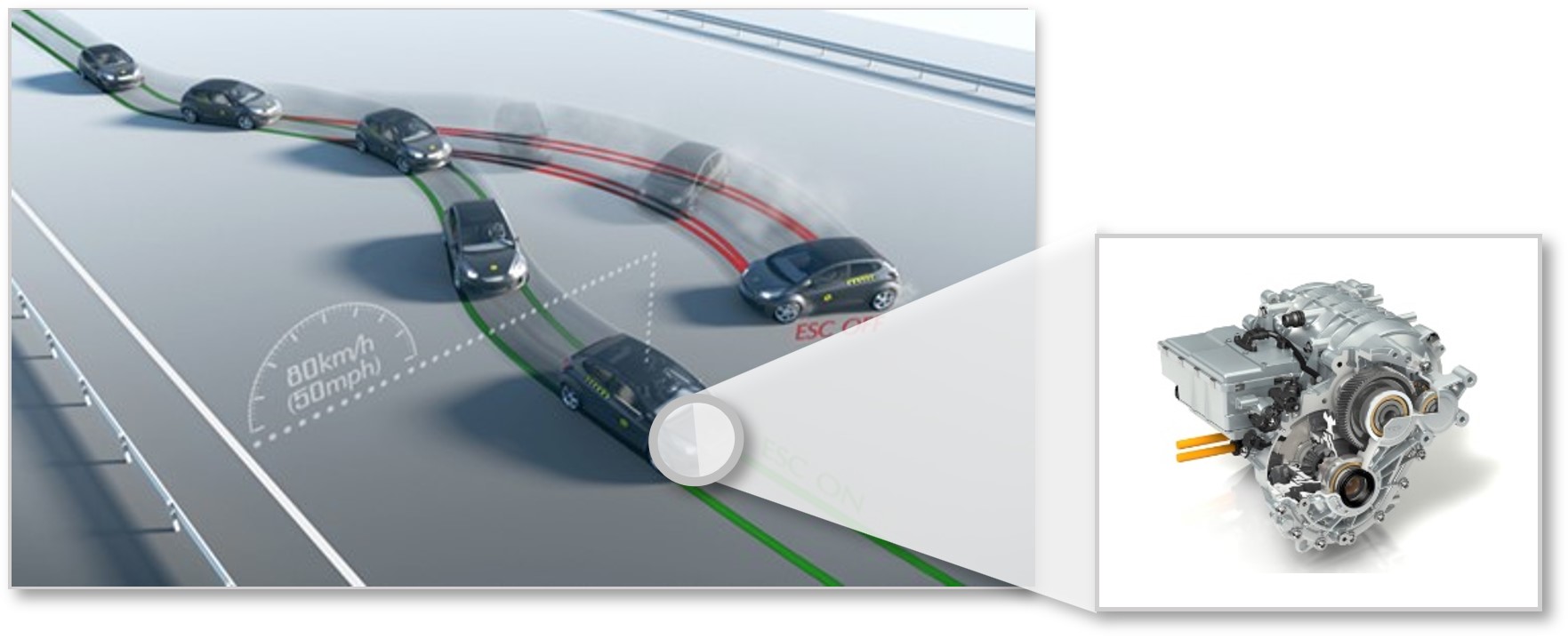
Figure 6. Electric machines used as traction motors in CAEVs
Furthermore, as the field of future mobility continues to evolve, the role of robot manipulators is also gaining prominence. Robot manipulators are now being utilized as charging devices for electric vehicles, and their usage is expected to become increasingly widespread. Looking ahead, mobile manipulators are poised to become vital platforms for logistics delivery and other mobility applications. Recognizing the significance of this development, our research at the MIC Laboratory extends beyond the domain of CAEVs to encompass the development of
advanced impedance control techniques for robot manipulators. By focusing on these techniques, we aim to achieve human-like robot operations and foster symbiotic human-robot interaction, propelling the field of future mobility toward new horizons.
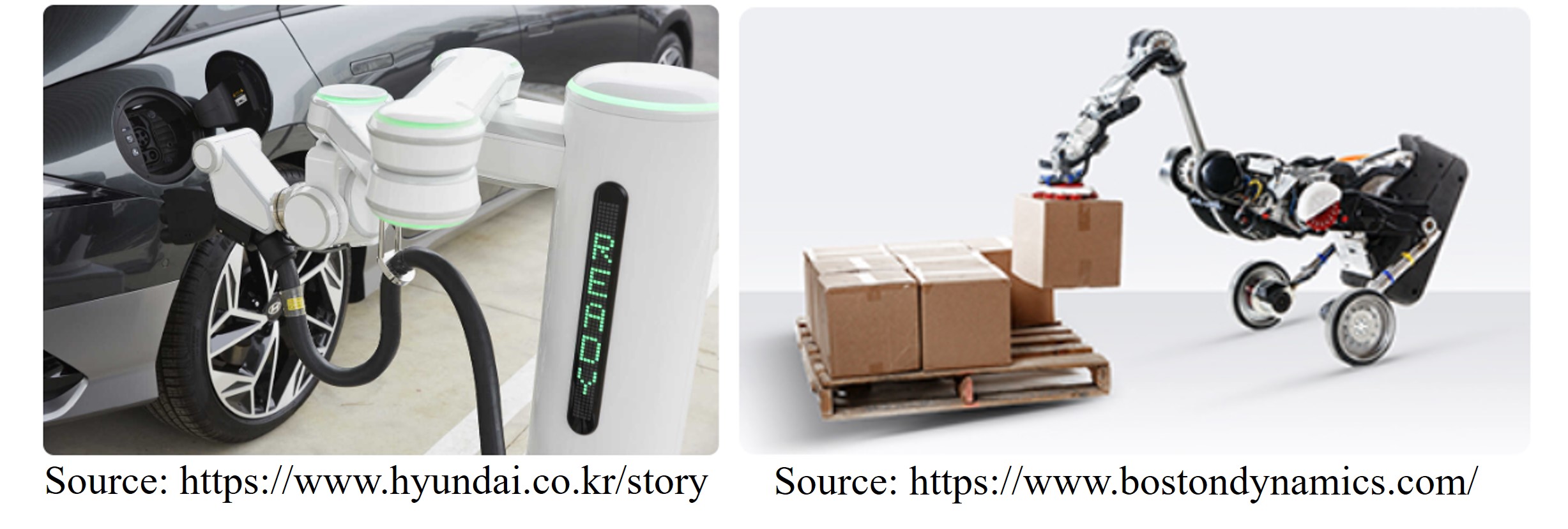
Figure 7. Usage of robot manipulators for future mobility
All the control problems we tackle involve solving complex optimal control problems characterized by mathematical complexity and uncertainties. Our research methodology entails
harnessing the synergies between control theories and artificial intelligence (AI) to develop innovative solutions. By leveraging the strengths of both disciplines, we strive to address these challenging problems in a comprehensive manner.

Figure 8. Control theories x AI
Through our interdisciplinary approach and collaborative efforts, the Mobility Intelligence and Control (MIC) Laboratory strives to revolutionize the control systems for future mobility, encompassing both CAEVs and robotic manipulators. By addressing the main problem and its associated subproblems, we aim to maximize efficiency, safety, and performance while paving the way for a sustainable and seamless transportation ecosystem. Join us in our mission as we shape the future of mobility and unlock the full potential of advanced control systems and robotics.